The Transformative Potential of Semantic Segmentation Video in Business
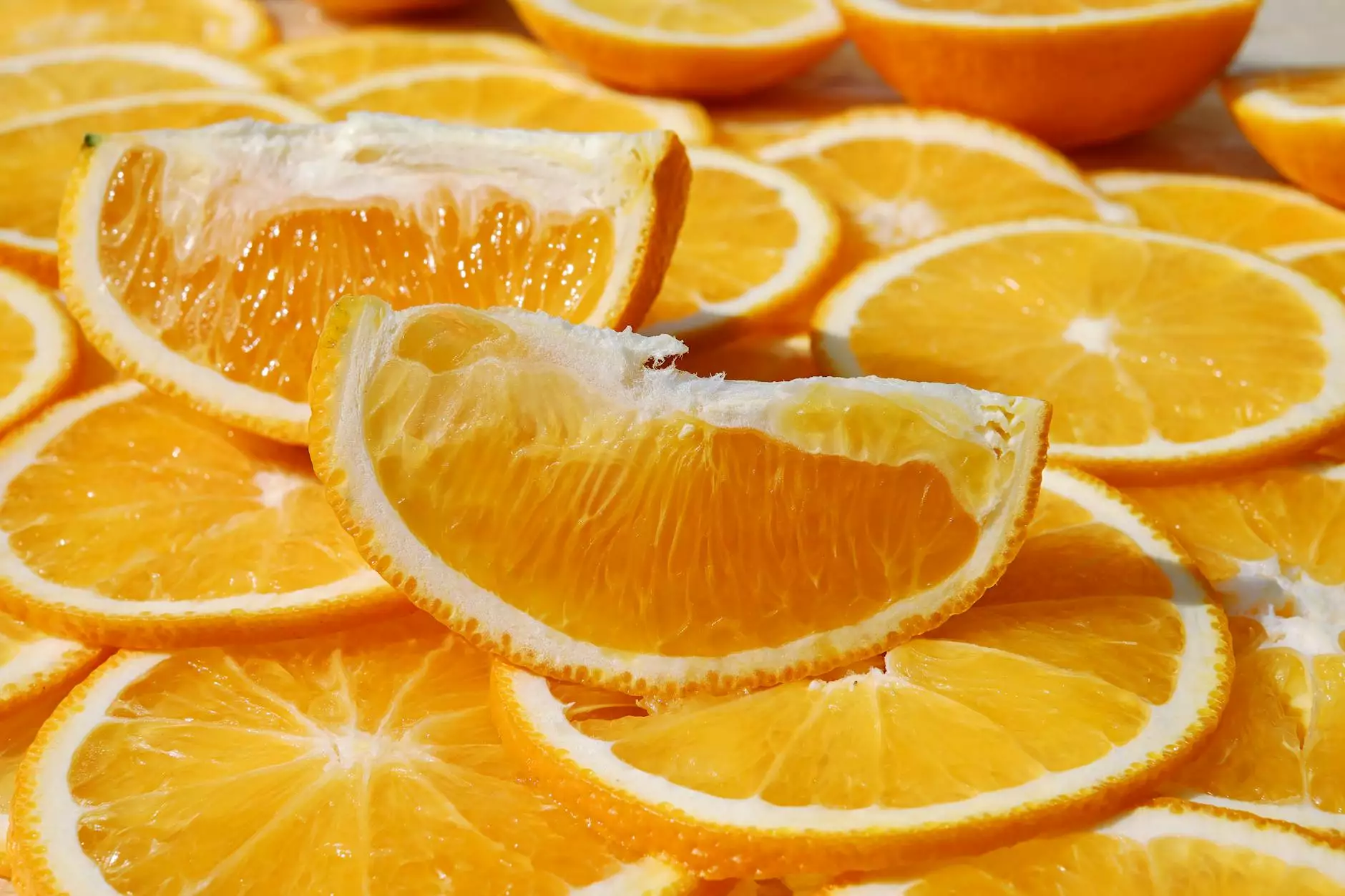
In today's fast-paced digital landscape, businesses are increasingly recognizing the significance of data-driven decisions. Among the various data analysis techniques, semantic segmentation video stands out as a revolutionary approach that allows companies to extract meaningful insights from visual content. In this comprehensive article, we will explore the intricacies of this technology, how it can enrich business operations, and the robust data annotation tools and data annotation platforms essential for implementing these solutions effectively.
Understanding Semantic Segmentation in Video
Semantic segmentation is a process that classifies each pixel in a video into predefined categories, enabling machines to understand the content of the video at a granular level. Unlike traditional object detection methods that merely identify and locate objects within an image or video frame, semantic segmentation goes a step further by providing context and meaning to each pixel.
This technique is particularly valuable in various sectors, including:
- Healthcare: Analyzing medical imaging to identify diseases.
- Autonomous Vehicles: Enhancing navigation systems by recognizing road signs, pedestrians, and other vehicles.
- Retail: Understanding customer behavior through video surveillance analysis.
- Agriculture: Monitoring crop health and identifying diseases through aerial imaging.
The Importance of Data Annotation in Semantic Segmentation
Data annotation is a critical step in preparing datasets for training machine learning models. For semantic segmentation video, accurate labeling of video content is essential to ensure that models learn effectively. This process involves tagging different segments of the video with corresponding labels, which provides the groundwork for the machine learning algorithms to make precise predictions.
Types of Data Annotation Tools
To facilitate effective data annotation, businesses can leverage various data annotation tools. These tools come equipped with features that streamline the annotation process, making it faster and more efficient. Some popular types include:
- Manual Annotation Tools: Allow human annotators to label the data rigorously.
- Automated Annotation Tools: Utilize AI and machine learning algorithms to automate parts of the annotation process.
- Collaborative Annotation Platforms: Enable teams to work together in real-time, improving accuracy and efficiency.
Key Features of an Effective Data Annotation Platform
The choice of a data annotation platform can significantly impact the quality of semantic segmentation video analysis. Here are key features to consider:
- User-Friendly Interface: A straightforward design that simplifies the workflow for annotators.
- Robust Annotation Tools: Customizable tools for drawing bounding boxes, polygons, and more for precise segmentation.
- Quality Assurance Mechanisms: Built-in checks and balances to ensure high labeling accuracy.
- Scalability: Ability to handle growing datasets and increased workloads as business demands grow.
- Integration Capabilities: Seamless compatibility with machine learning frameworks and other business tools.
Applications of Semantic Segmentation in Business
The applications of semantic segmentation video are diverse and can lead to innovative solutions and enhanced operational efficiencies across many industries. Here are some notable examples:
1. Enhancing Customer Experience in Retail
Retail businesses are increasingly using video analytics powered by semantic segmentation to monitor customer behavior within stores. By understanding how customers interact with products, businesses can optimize store layouts, improve product placements, and ultimately enhance the overall shopping experience.
2. Improving Safety in Autonomous Vehicles
In the automotive industry, semantic segmentation is essential for the development of autonomous driving technology. Vehicles equipped with advanced cameras can interpret their surroundings by segmenting video feeds into actionable insights. This allows for safer navigation by identifying pedestrians, cyclists, and road conditions in real time.
3. Streamlining Operations in Manufacturing
Manufacturers are using semantic segmentation to monitor production processes and ensure quality control. By analyzing video footage from production lines, businesses can detect defects, optimize machine performance, and maintain consistent output quality.
4. Revolutionizing Healthcare Diagnostics
In the healthcare sector, semantic segmentation video is transforming diagnostics. By accurately segmenting and analyzing medical images, healthcare professionals can improve disease detection and patient outcomes. For example, semantic segmentation techniques can identify tumors in radiology images, enabling earlier interventions.
How to Implement Semantic Segmentation Video in Your Business
Implementing semantic segmentation video involves several steps, each crucial to ensuring success. Below is a structured approach to adopting this technology:
Step 1: Define Your Objectives
Clearly articulate what you aim to achieve with semantic segmentation video. Whether it's enhancing customer experience or improving safety in autonomous systems, defining your goals is the first step toward successful implementation.
Step 2: Choose the Right Data Annotation Tool
Investing in an effective data annotation tool is key. Evaluate various options based on your specific use case, scalability needs, and budget. Consider platforms that offer robust features and a user-friendly interface.
Step 3: Collect and Prepare Your Data
Gather video data relevant to your objectives. Ensure that your data is diverse and representative of the scenarios you want to analyze. Preprocessing the data may involve enhancing video quality and converting formats as needed.
Step 4: Annotate Your Data
Utilize your chosen annotation tool to label the collected video data accurately. Engage skilled annotators who understand the nuances of semantic segmentation. This step is vital, as quality annotations directly impact the performance of the machine learning model.
Step 5: Train Your Model
With annotated data in hand, you can proceed to train your machine learning model. Employ the appropriate algorithms that best fit your use case. Frequent evaluation and fine-tuning will enhance your model’s performance.
Step 6: Deploy and Monitor
Once your model is trained, deploy it into your business processes. Continuously monitor its performance and make adjustments as necessary. Regular updates and retraining with new data will help improve accuracy over time.
Challenges and Considerations in Semantic Segmentation
While the benefits of semantic segmentation video are numerous, businesses must be aware of potential challenges:
- Data Quality: High-quality, diverse datasets are crucial for effective training.
- Complexity of Implementation: The process can be resource-intensive, requiring investment in technology and skilled personnel.
- Ethical Considerations: Issues related to privacy and surveillance must be managed carefully, particularly in sectors like retail and healthcare.
Conclusion: The Future of Semantic Segmentation Video in Business
The integration of semantic segmentation video into business operations promises to revolutionize how organizations analyze and interpret visual data. By leveraging advanced data annotation platforms and tools, businesses can unlock valuable insights, drive innovation, and enhance customer experiences.
As technology continues to evolve, the applications of semantic segmentation will expand, opening new avenues for operational optimization and strategic decision-making. Embracing this technology now will position your business as a forward-thinking leader in the digital age.
For those looking to dive deeper into the power of semantic segmentation video and explore cutting-edge tools, visit keylabs.ai—your partner in unlocking the full potential of data annotation.